There is a lot of hype about Artificial Intelligence (AI) at the moment – from its potential benefits to the risks it poses in some areas. AI is already being used across multiple sectors, including diagnostics and healthcare, to analyse images and data, identifying patterns across large datasets. This helps detect diseases more quickly and, in some cases, at an earlier stage, as well as assisting in the development of new treatments.
However, AI is still in its infancy, and its full potential remains largely untapped. Like AI itself, we are still learning. Over the past year, there has been a surge in software companies promoting AI as part of their solutions. Yet, it can be difficult to determine whether these features are based on generative AI or traditional AI. Generative AI enables a system to process vast amounts of data, understand it, and learn from it as a human would—creating new ideas and content. Traditional AI, on the other hand, is typically programmed by humans to automate processes, handle data entry, and generate analytics based on pre-defined parameters.
Regardless of the type of AI in question, one thing is certain: AI relies on data. And data which needs to be good, high-quality data. The old adage ‘rubbish in, rubbish out’ has never been more relevant.
What is an AI strategy?
Like all businesses, laboratories are increasingly exploring AI: where it can be used, how it can be integrated, and the benefits and costs associated with its implementation.
With the rise of computational power and sophisticated algorithms, AI is enhancing data analysis, streamlining workflows, and improving decision-making processes in laboratories. However, implementing AI effectively requires a well-defined strategy.
The first step in developing an AI strategy is identifying the specific benefits you aim to achieve, how AI aligns with your laboratory’s business objectives and how, or if, you can leverage your existing technology and systems, such as LIMS. Are you looking to boost productivity? Enhance innovation? Automate routine processes? Improve data accuracy and decision-making?
Key considerations
- Data readiness: AI algorithms rely on vast amounts of high-quality data to function effectively. Laboratories need to assess whether their data is standardised, structured, and readily accessible for AI applications.
- Infrastructure: Implementing AI requires the right computational infrastructure, such as cloud-based solutions or high-performance computing systems, to process large datasets efficiently.
- Integration with existing systems: AI should complement and enhance existing laboratory workflows rather than disrupt them. Integration with current laboratory management systems is crucial.
- Regulatory compliance: Laboratories must ensure that AI implementation adheres to industry regulations and data security standards to maintain compliance and protect sensitive information.
- Training and adoption: AI-driven systems require trained personnel who can interpret AI-generated insights and integrate them into laboratory decision-making.
AI also introduces cybersecurity challenges. Sensitive laboratory data could be more exposed to exploitation, and AI systems themselves are vulnerable to adversarial attacks. These attacks involve manipulating input data to mislead AI models, potentially leading to incorrect or harmful outputs.
To mitigate such risks, laboratories must adopt a proactive, multi-layered security approach. Strong encryption for data in transit and at rest ensures protection against unauthorised access. Implementing robust authentication measures, such as multi-factor authentication (MFA), is critical in securing system access and preventing breaches.
Where a LIMS supports your AI strategy
A Laboratory Information Management System (LIMS) plays a crucial role in supporting an AI strategy and its adoption within laboratories. Many modern LIMS platforms already incorporate traditional AI features, such as:
- Conducting complex calculations.
- Consolidating data by connecting systems and equipment.
- Visualising data through analytics dashboards.
- Mapping standard operating procedures (SOPs) and processes to streamline operations.
Additionally, LIMS can support the integration of generative AI by:
- Standardising data to improve quality and integrity.
- Centralising and consolidating data from various sources.
- Providing secure RESTful APIs to connect with generative AI applications.
- Enhancing data governance to ensure AI-driven decisions are based on accurate and reliable information.
- Facilitating predictive analytics by feeding AI models with structured and comprehensive data to forecast trends and outcomes.
- Improving compliance and audit trails by ensuring that AI-generated insights are well-documented and traceable.
A well-implemented LIMS ensures that laboratories maintain structured, high-quality data—laying the groundwork for AI to function effectively. This not only enhances operational efficiency but also positions laboratories to leverage AI innovations responsibly and securely.
Future trends in AI and LIMS
As AI technology continues to evolve, laboratories leveraging a robust LIMS will be in a prime position to take advantage of emerging trends, including:
- Automated decision-making: AI-driven LIMS will be able to recommend optimal laboratory processes, improving efficiency and accuracy.
- Machine learning for quality control: Advanced AI models can detect anomalies and deviations in test results, enhancing quality control measures.
- Personalised medicine applications: AI-powered LIMS may assist in tailoring treatments based on individual genetic data and biomarkers.
- Real-time monitoring and alerts: AI-enabled LIMS can proactively alert scientists to potential issues in lab processes, reducing errors and downtime.
As AI integration within laboratories advances, the importance of a well-structured LIMS will continue to grow. The combination of AI capabilities with a comprehensive LIMS solution will create more efficient, secure, and innovative laboratory environments.
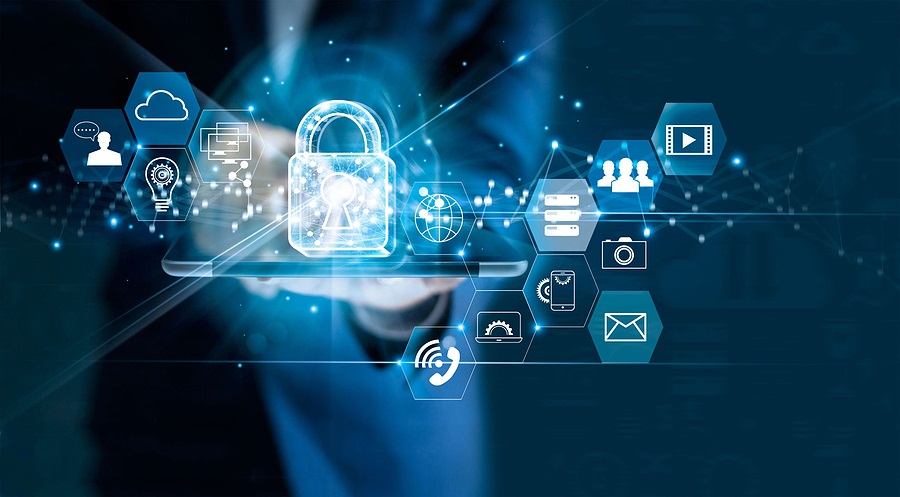
We will look at how LIMS features and tools available as well as how the emerging use of AI impacts cybersecurity.
Comments are closed.